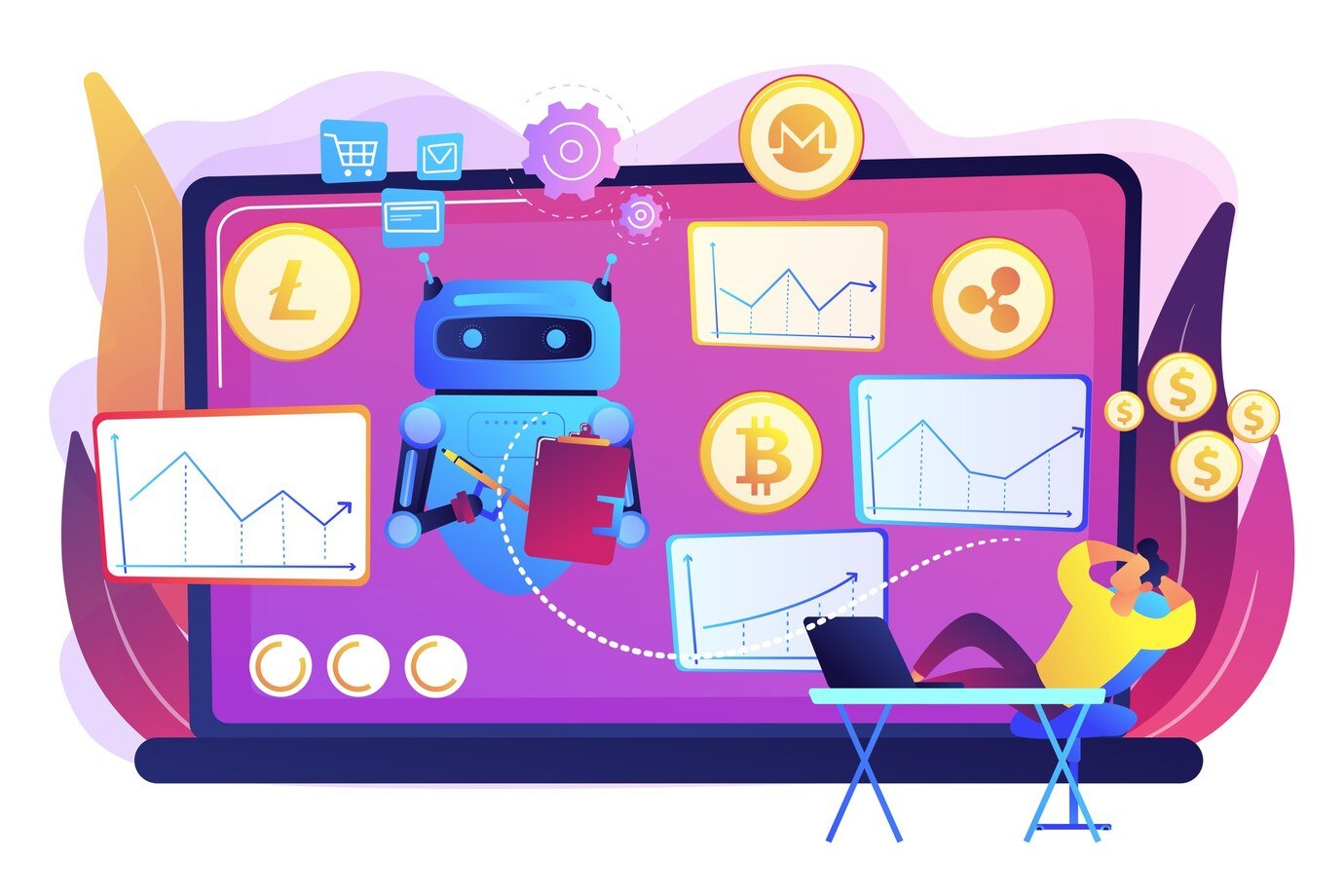
The Role of Machine Learning in Predictive Banking
Posted on April 7, 2023
Machine learning (ML) plays a pivotal role in transforming the banking industry by enabling predictive analytics, which allows financial institutions to make smarter, data-driven decisions. Here’s a breakdown of how machine learning contributes to predictive banking:
1. Credit Scoring and Risk Management
Machine learning algorithms analyze a vast array of data points beyond traditional credit scoring models, including transaction history, social media activity, and purchasing behavior. By doing so, they provide more accurate and personalized credit scores, allowing banks to assess risks better and make informed lending decisions. This predictive model also helps identify potential loan defaults before they happen.
2. Fraud Detection and Prevention
Machine learning models can analyze patterns in transaction data in real time, identifying irregularities that may suggest fraudulent activity. By recognizing these anomalies early, banks can prevent or minimize losses due to fraud. These systems continuously improve as they process more data, enhancing their ability to spot emerging threats.
3. Customer Retention and Engagement
Predictive analytics powered by ML helps banks understand customer behavior and identify signs of churn. By analyzing customer transactions, interactions, and preferences, banks can predict when customers might leave and implement targeted retention strategies. Additionally, banks can deliver personalized offers and products tailored to individual customer needs, improving engagement and satisfaction.
4. Market Trend Prediction
Machine learning algorithms analyze historical data, news, and market signals to forecast market trends and asset prices. This enables banks to provide more accurate investment advice, adjust portfolios proactively, and mitigate risks, all while staying ahead of market movements.
5. Operational Efficiency
Predictive models help banks optimize their internal processes. For example, they can predict the volume of customer queries, enabling better allocation of resources. ML also helps in automating routine tasks such as data entry and compliance checks, reducing operational costs and improving service speed.
6. Loan Default Prediction
By analyzing various factors, including economic indicators and borrower behavior, machine learning models predict the likelihood of loan defaults. These predictive models allow banks to take preventive actions, such as adjusting interest rates or renegotiating loan terms before defaults occur.
7. Portfolio Management
Machine learning assists in the analysis of diverse assets within a portfolio to predict their future performance based on historical trends, correlations, and external factors. This enables banks and financial institutions to make better investment decisions and optimize their portfolios for maximum returns.
8. Personalized Banking Products
ML enables banks to personalize products and services based on individual customer needs, behavior, and preferences. Predictive models help determine what services, such as savings accounts, credit products, or investment options, would be most beneficial for each customer, driving revenue and enhancing customer satisfaction.
By leveraging machine learning in predictive banking, financial institutions can make more accurate, efficient, and personalized decisions, ultimately improving profitability, reducing risk, and enhancing customer experiences.
Categories: Banking